How Do Financial Analysts Overcome Significant Challenges in Data Analysis?
FinancialAnalysis.io

How Do Financial Analysts Overcome Significant Challenges in Data Analysis?
Overcoming significant challenges in financial analysis can be daunting, but finance experts have shared their strategies to tackle these obstacles. This article reveals how upgrading a Salesforce implementation can significantly improve data management. Additionally, it highlights the importance of implementing robust data governance policies to ensure data integrity and consistency. Discover seven invaluable insights from finance professionals who have successfully navigated the complexities of financial data analysis.
- Upgrade Your Salesforce Implementation
- Standardize Data and Collaborate
- Use Predictive Modeling
- Detect Anomalies with Machine Learning
- Utilize Data Visualization Tools
- Collaborate with Domain Experts
- Implement Robust Data Governance Policies
Upgrade Your Salesforce Implementation
During my time at N26, I encountered a particularly challenging situation while working in banking operations. We needed to streamline our garnishment and insolvency processes, but the data was scattered across multiple systems and formats. I remember spending long hours diving into Salesforce, our CRM tool, trying to make sense of thousands of customer records and financial transactions. The real breakthrough came when I developed a new approach to upgrade our Salesforce implementation, creating custom workflows that could track and analyze these complex cases more efficiently. Now at Spectup, I use this experience to help startups build robust financial-monitoring systems. One recent example was working with a fintech startup that struggled with their financial reporting—we implemented similar principles I learned at N26 to create a clear, actionable financial dashboard that helped them secure their Series A funding.
The key lesson I took from these experiences is that financial data challenges often require a combination of technical knowledge and practical business understanding. This balanced approach has become a cornerstone of how we at Spectup help startups prepare their financial narratives for investors.
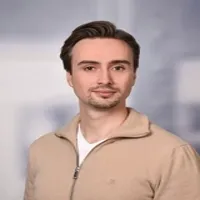
Standardize Data and Collaborate
My biggest difficulty in financial analysis included inconsistent and incomplete data for an acquisition deal. I saw this issue and, to fix it, I started gathering all the data into a single source and bringing the formats to the same standard, which helped me identify the wrong data and ignore the mutual concepts. Where I did not have data readily available, I adhered to the industry benchmarks and historical company data, creating reasonable and well-documented assumptions and making sure that this was well-communicated to the stakeholders. I worked with various teams, including accounting and operations, to get context beyond the figures. Finally, I conducted a sensitivity analysis to show what the outcome would look like under various sets of assumptions, from which we learned that the large data gaps nevertheless could be assessed for risk during the gap period. This not only allowed for a robust analysis but also provided me valuable experience in needing structure, collaboration, and transparency in any complicated finance work.
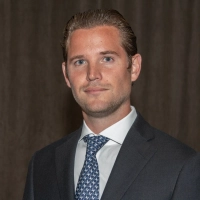
Use Predictive Modeling
Financial analysts use predictive modeling to make forecasts about future trends and assess potential risks that might arise. By analyzing past data and identifying patterns, they are able to predict future market behavior. This approach provides a way to prepare for and mitigate risks before they become significant issues.
Through these predictions, businesses can make more informed decisions to navigate market uncertainties. To gain maximum benefit, companies should invest in training and technology for predictive modeling tools.
Detect Anomalies with Machine Learning
Machine learning algorithms are frequently utilized by financial analysts to automatically detect anomalies in large datasets. These algorithms can quickly and accurately identify unusual patterns that might indicate potential issues or fraud. With machine learning, the data analysis process becomes more efficient and reliable, reducing the manual effort required.
This not only saves time but also enhances the accuracy of the findings, ensuring no critical details are missed. Organizations should continue to integrate advanced machine learning solutions to streamline their analytical processes.
Utilize Data Visualization Tools
Data visualization tools are key for financial analysts to present complicated data in a clear and concise manner. These tools transform complex datasets into visual formats such as graphs and charts, making it easier to understand trends and insights at a glance. This helps stakeholders quickly grasp the financial status and make informed decisions.
By presenting data visually, analysts can effectively communicate their findings and recommendations. Businesses should encourage the use of innovative data visualization tools to improve data comprehension among decision-makers.
Collaborate with Domain Experts
Financial analysts often collaborate with domain experts to better understand the context and implications of financial data. Domain experts provide invaluable insights that help make sense of raw data, making it easier to draw meaningful conclusions. This collaboration ensures that the data is interpreted accurately, leading to more reliable and actionable analysis.
By working together, analysts and experts can tackle complex financial challenges more efficiently. Companies should foster strong partnerships between analysts and domain specialists to enhance the quality of their data analysis.
Implement Robust Data Governance Policies
Implementing robust data governance policies is crucial for financial analysts to ensure data accuracy and reliability. These policies establish clear guidelines for data management, including data collection, storage, and usage practices. By adhering to these policies, analysts can maintain the integrity of the data they work with, minimizing the risk of errors and inaccuracies.
Reliable data governance creates a trustworthy foundation for all subsequent analysis. Organizations should prioritize the development and enforcement of comprehensive data governance frameworks to support effective data analysis.